Modern society has been relying more and more on engineering advance of autonomous systems, ranging from individual robots (such as a robotic arm for manufacturing, a self-driving car, or an autonomous vehicle for planetary exploration) to cooperative ones (such as a human-robot team, swarms of drones, etc).
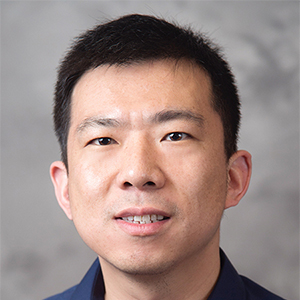
Dr. Shaoshuai Mou,
Purdue University
In this talk we will present our most recent progress in developing a fundamental framework for learning and control in autonomous systems. The framework comes from a differentiation of Pontryagin’s Maximum Principle and is able to provide a unified solution to three classes of learning/control tasks, adaptive autonomy (i.e. optimal control adaptive to additional objective function), inverse optimization (or learning from demonstrations in robotics), and system identification. An extension of this framework to incorporate hard constraints for safety will also be described. We will also present applications of this framework into human-robot teaming, especially in enabling an autonomous robot to take guidance from human operators, which is usually sparse and vague, and also adapt robot’s performance incrementally from human’s corrections.
Dr. Shaoshuai Mou is the Elmer Bruhn associate professor in the School of Aeronautics and Astronautics at Purdue University. He received a Ph.D. in Electrical Engineering at Yale University in 2014 under the supervision of Prof. A. Stephen Morse. He worked as a postdoc researcher at MIT in 2014, and then joined Purdue University as a tenure-track assistant professor in Aug. 2015.
His research group Autonomous & Intelligent Multi-agent Systems (AIMS) lab has been focusing on advancing control theories with recent progress in optimization, networks and machine learning for autonomous and robotics systems, with particular research interest in distributed algorithms for control, optimization and learning for multi-agent systems, inverse optimal control for robotics, parameter adaptation in optimal control, integration of learning and control, human-robot teaming, etc.