How to transfer the successes of machine learning in artificial environments such as video games and language modelsto real-world physical systems is one of the major open questions for the coming decades. Addressing this challenge requires overcoming several obstacles, including safety concerns arising from model mismatches and the scarcity of real-world data.
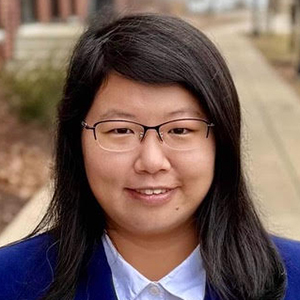
Dr. Yingying Li,
UIUC – Illinois
In this talk, we focus on the two key challenges mentioned above: safety and limited data. We explore two complementary approaches—a model-based and a model-free one—because we believe both are crucial for tackling this open problem. Part 1 of the talk focuses on the model-based approach, specifically our recent results on system identification (ID). In particular, we establish non-asymptotic convergence rates for linearly parameterized nonlinear systems, addressing a long-standing open question in nonlinear system ID. These system ID results can be applied to robust adaptive control, enabling less conservative control design while ensuring constraint satisfaction. Part 2 of the talk introduces a model-free framework. Specifically, we present an online switching control algorithm that guarantees stability and sublinear regret during learning, even without knowledge of a specific stabilizing controller. Finally, we highlight how our model-free approach in Part 2 can be naturally integrated with the model-based approach in Part 1
Yingying Li is an assistant professor in the Department of Industrial and Enterprise Systems Engineering and the Coordinated Science Laboratory (CSL) at the University of Illinois Urbana-Champaigne (UIUC). She is also affiliated with the Department of Electrical and Computer Engineering and the Department of Mechanical Science and Engineering.
Her research interests are at the intersection of control, machine learning, reinforcement learning, and optimization, with applications in smart grids, smart cities, and robotics. Her work was selected as the Editor’s Choice by Automatica, and she was recognized as a Rising Star in Cyber-Physical Systems in 2022.
Yingying received her Ph.D. and master’s degree in applied mathematics from Harvard University, in addition to a bachelor’s degree in applied mathematics from the University of Science and Technology of China. She also spent time at the Department of Computing & Mathematical Sciences at the California Institute of Technology, Coordinated Science Laboratory (CSL) at UIUC, and the MIT-IBM Watson AI Lab.