Monolithic microelectronic design is facing tremendous challenges in the growing need of memory bandwidth, latency, and energy efficiency. Although recent advances (e.g., domain-specific acceleration, near-memory and in-memory computing techniques) try to address these issues, the scaling trend of monolithic design still lags behind the ever-increasing demand of AI algorithms and other data-intensive applications.
In this context, technological innovations, particularly 2.5D/3D integration through packaging and monolithic methods, are critical to enabling heterogeneous integration (HI) and bringing significant performance, energy and cost benefits beyond traditional chip design.
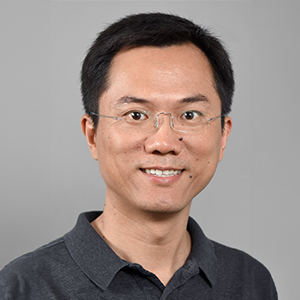
Such a paradigm shift requires a tight collaboration between packaging and chiplet design through the entire design cycle. In this context, we will present our performance benchmark tool to explore heterogeneous integration. This simulator models device/circuit/network/architecture to address the challenges in hardware-algorithm co-design. Leveraging the new tool, we will demonstrate two heterogenous innovations, the big-little chiplet architecture and a 65nm test chip for 3D in-sensor computing. We will conclude this talk with brainstorming on the potential and research needs of future 3D integration.
Yu Cao received his doctoral degree in electrical engineering from University of California, Berkeley, in 2002. He is now a professor in the Department of Electrical and Computer Engineering at the University of Minnesota. Before joining UMN, he was a professor of electrical engineering at Arizona State University.
He has published numerous articles and two books on nano-CMOS modeling and physical design. His research interests include neural-inspired computing, hardware design for on-chip learning, and reliable integration of nanoelectronics.
Prof. Cao is a Distinguished Lecturer of the IEEE Circuits and Systems Society. He was a recipient of the 2020 Intel Outstanding Researcher Award, the 2009 ACM SIGDA Outstanding New Faculty Award, the 2006 NSF CAREER Award, the 2006 and 2007 IBM Faculty Award, and five Best Paper Awards. He is a Fellow of the IEEE.